Data Wrangling: Strategies for Cleaning and Preparing Data for Analysis
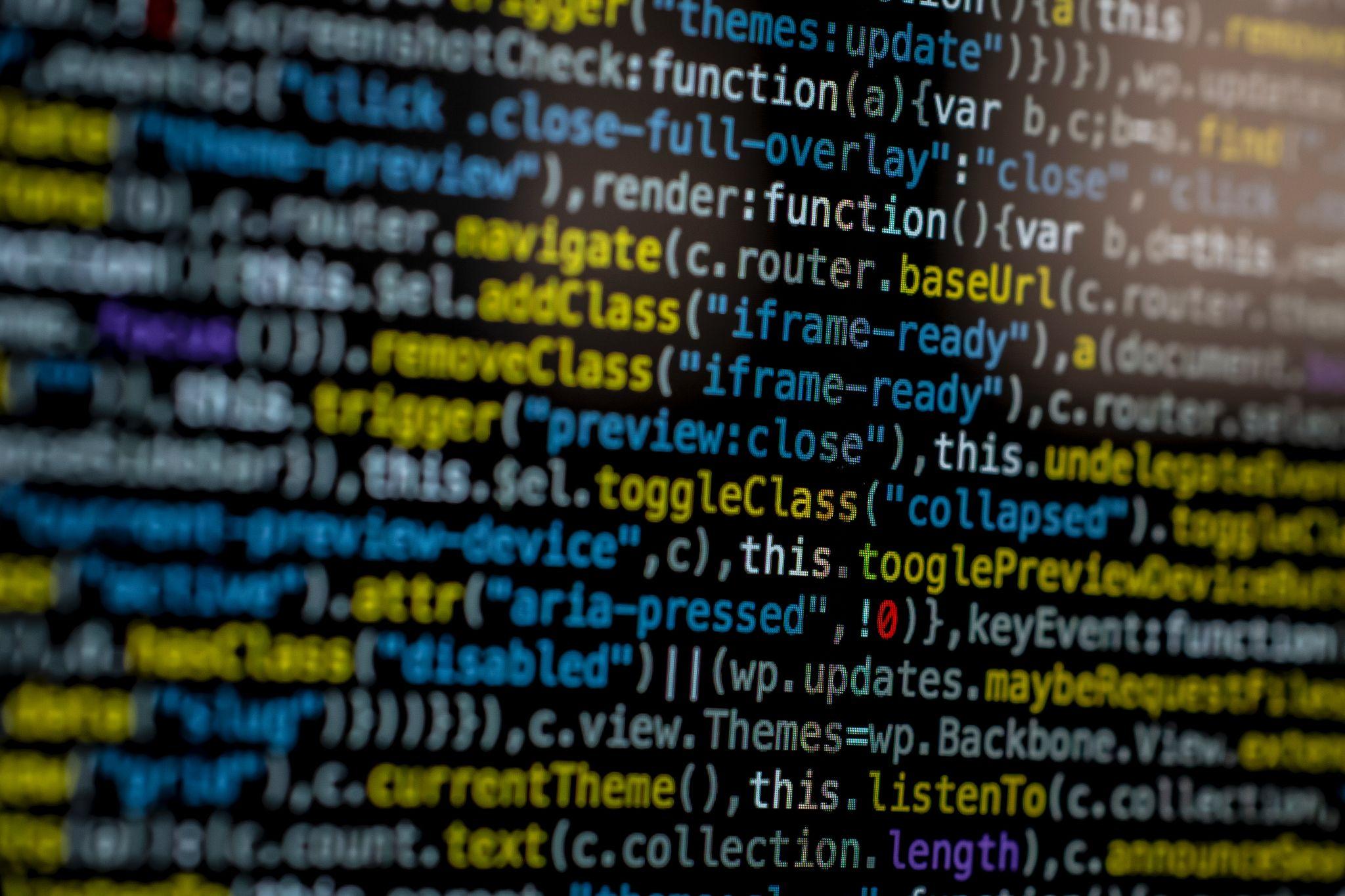
In the contemporary age of information, we find ourselves awash in a sea of data. Each data point, akin to a droplet in this vast ocean, holds the potential to illuminate decision-making processes and drive an organisation’s success. The key to unlocking this latent value from data is the process of data wrangling. This crucial process involves the transformation of raw data into a form that is more suitable for analysis and insight generation. However, the process can often be a daunting task for many. Thus, a comprehensive understanding and a structured approach are vital for effective data wrangling.
Unpacking the Concept of Data Wrangling
Data wrangling, often an overlooked aspect in the data analysis process, could be the deciding factor between the success and failure of a project. It primarily involves identifying discrepancies in data, cleaning it, and subsequently transforming it into a more valuable form. This newly transformed data can be readily used for business intelligence and other analytical processes.
The techniques employed in data wrangling broadly encompass three critical processes: data cleansing, data transformation, and data enrichment. Data cleansing is the process of detecting and correcting or removing errors, inconsistencies, and inaccuracies from datasets. This process ensures that the data is reliable and valid, serving as a solid foundation for further steps in data analysis.
On the other hand, data transformation is the conversion of data from one format or structure into another, more digestible one. The aim of this process is to prepare data in such a way that analytical tools can extract meaningful insights from it.
Data enrichment completes the data wrangling process. This process aims to enhance raw data with additional, context-providing information. This enriched data offers a more comprehensive view of the phenomena being studied, thereby leading to more precise and valuable insights.
Essential Strategies for Effective Data Wrangling
- Identify and Understand the Data
The first step to effective data wrangling is to develop a deep understanding of the data at hand. This initial understanding involves identifying the source of data, understanding its structure, and becoming familiar with the variables within the dataset.
Identifying the source of data allows us to gauge its reliability. Reliable data is essential for credible and dependable results. Thus, data should always be collected from reliable and trusted sources. The structure of the data informs us about the type of data wrangling techniques we should employ. For instance, structured data might require a different approach as compared to unstructured data. Understanding the relevant variables within the dataset allows us to direct our data wrangling efforts efficiently, focusing on aspects of data that matter most to the study.
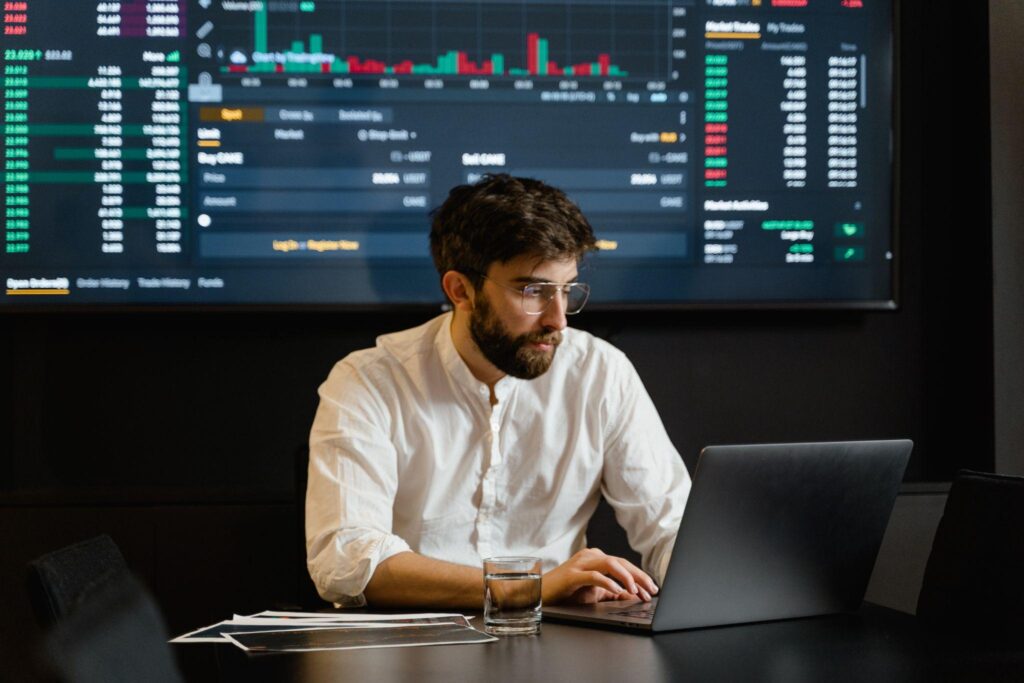
- Data Cleaning
The next step in the data wrangling process is data cleaning. Data cleaning is crucial for ensuring the quality and reliability of the data, which directly affects the accuracy of the subsequent analysis.
Handling missing values is the first task in the data cleaning process. Missing data can introduce a significant amount of bias, making the analysis unreliable. Several strategies can be employed to tackle missing data, ranging from data imputation methods to discarding data, based on the nature and extent of missing data.
Next in line is the correction of inconsistent or incorrect data. Inconsistencies or incorrect data can be present in many forms, including data in wrong units, typographical errors, or even wrong data types. These errors should be corrected to ensure the integrity of the data.
Duplicate data is another factor that can distort the results of the analysis. Therefore, duplicates need to be identified and removed. Outlier treatment is the final step in the data cleaning process. While some outliers can provide valuable insights, others are often errors that need correction.
- Data Transformation
Once the data is cleaned, the data transformation process can commence. This process involves modifying data into a format that is suitable for analysis. Depending on the requirements of the analysis, this could include several processes.
One common process in data transformation is the merging of data from different sources or tables. Merging data allows us to have all relevant information in one place, making the analysis process more streamlined.
Creating new variables that can provide additional insights is another important aspect of data transformation. For instance, from a dataset with date and time of purchases, we can create new variables such as ‘day of the week’ or ‘time of the day’ that might provide more nuanced insights.
Rescaling variables to a standard scale and one-hot encoding for categorical variables are other transformations that are often required. These transformations make the data more amenable to various analytical methods.
- Automate the Process
Automation is the future of data wrangling. Developing scripts or using tools to automate tasks that are repeated across datasets can save a significant amount of time and resources. Additionally, automation reduces the chance of errors that might creep in while performing manual data wrangling.
Automated data wrangling can range from simple scripts that clean data in specific ways to complex systems that use machine learning to automate a significant portion of the data wrangling process. While the initial set up of these systems might require time and expertise, the time saved in the long run more than makes up for it.
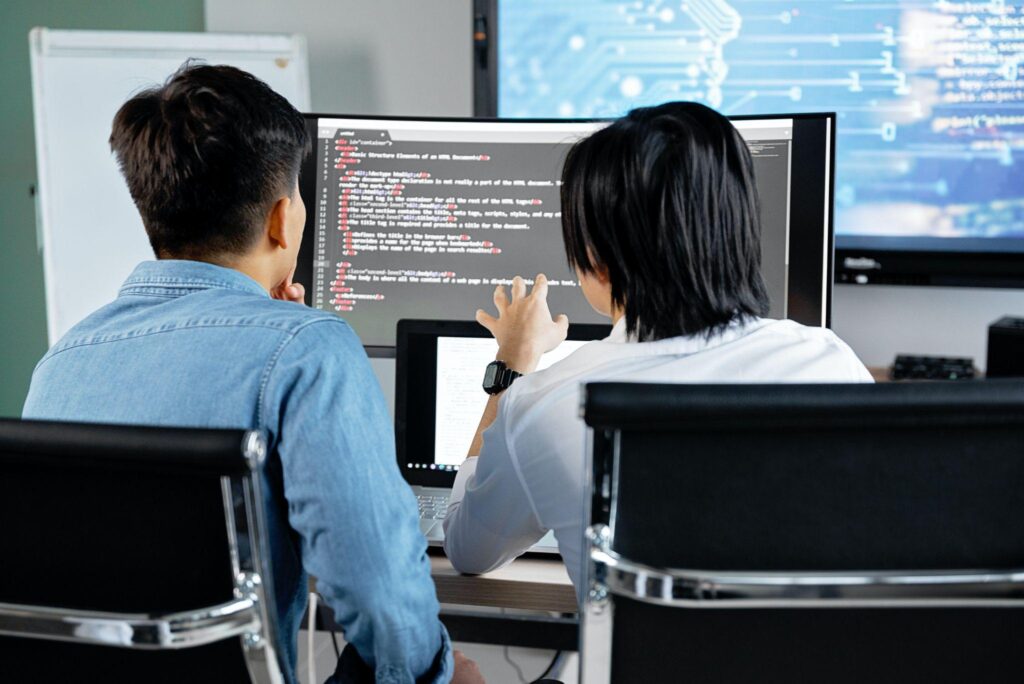
- Data Verification
Once the data has been wrangled, it is essential to verify it. Data verification involves ensuring that the data transformation process has been carried out correctly and is ready for analysis.
Verification could include several processes such as checking for outliers, validating data against external sources, or using statistical methods to confirm the reliability of the data. It is an essential step that ensures the integrity of the data and the subsequent analysis process.
Empower Your Data Journey
While data wrangling can often be a complex and time-consuming process, it is a necessary step to extract meaningful insights from data. Mastering this process could be the difference between a successful analysis and a failed one.
However, if the process feels overwhelming or if you simply desire expert help to ensure that you’re making the most of your data, we at Pangaea X are here to assist. We connect businesses with top data freelancers from around the globe. Whether you need support in data wrangling, data analytics, or any other facet of data science, our platform can help you find the right expert for your needs. Explore the wealth of talent available at Pangaea X today, and let us help you transform your data into a powerful tool for your success.
Get your data results fast and accelerate your business performance with the insights you need today.