Top 7 Data Analytics Trends for 2022
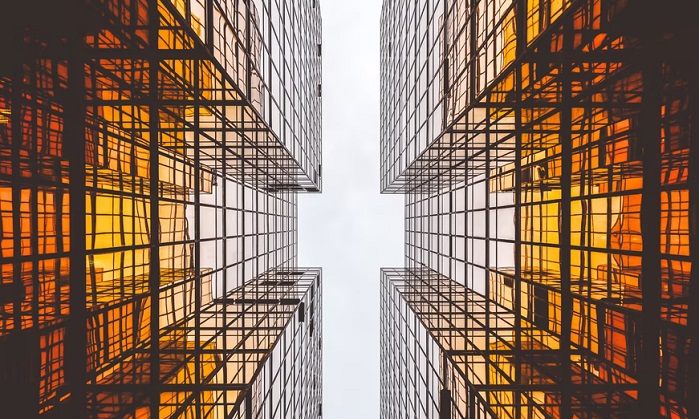
Businesses generate huge amounts of data. Our traditional ways of understanding and interpreting data just can’t provide the needed insights to support business decisions today. Covid-19 has changed so many things, but one of the most long-lasting impacts of the pandemic will be how it has accelerated digital transformation.
As we go about our daily lives, we are generating ever growing amounts of data. If you are a small business owner or a business manager, you need to know about the latest data analytics trends that can impact you. Some of these trends have been around for a while, but in 2022, we expect to see them begin to have a greater impact with the development of many more real-life applications.
1. AutoML
Automated machine learning (AutoML) is an exciting trend that refers to the process of automating the tasks of applying machine learning to real world problems.
AutoML tools try to make machine learning accessible for non-machine learning experts. The goal is to allow anyone to apply machine learning through simple, easy to use interfaces to automate data cleansing and preparation. In the long-term, AutoML will also help in building models and creating algorithms and neural networks.
For you, this means that you can spend less time worrying about how the data is going to be cleaned up and arranged, and more time focusing on identifying business issues and solutions.
2. Deepfakes, generative AI and synthetic data
Generative network technology involves creating realistic synthetic data. In the case of deepfakes, that means audio or video that can fool human viewers. Although there is concern about the malicious use of this ability, this technology has many positive potential uses, for instance, synthetic human faces can be used to train facial recognition algorithms for medical applications. It could be used to provide better automated customer service or be used to improve on-line teaching and training.
Generative AI could also be used to create text-to-speech functions to allow designers to create conceptual images simply through verbal descriptions.
3. Small data and TinyML
We often talk about “Big Data” to refer to the huge amount of digital data that is being generated, collected and analysed. But it’s not just the data that is big. But the ML (machine learning) algorithms being used to process this data is also enormous. One common example given is that of GPT 3 – the largest and most complex system for modelling human speech which involves around 175 parameters.
But what happens when you are not operating on a cloud-based system with unlimited bandwidth? That’s when small data and TinyML come in.
TinyML describes machine learning algorithms designed to take up as little space as possible so that they can be run on underperforming hardware close to where it is going. TinyML can provide AI capabilities to a wide range of devices helping to make them more secure, energy efficient and adaptable. TinyML applications will soon start appearing in cars, wearables, home appliances, industrial and farm equipment and so on, helping to make many aspects of our lives more convenient.
4. Data-driven customer experience
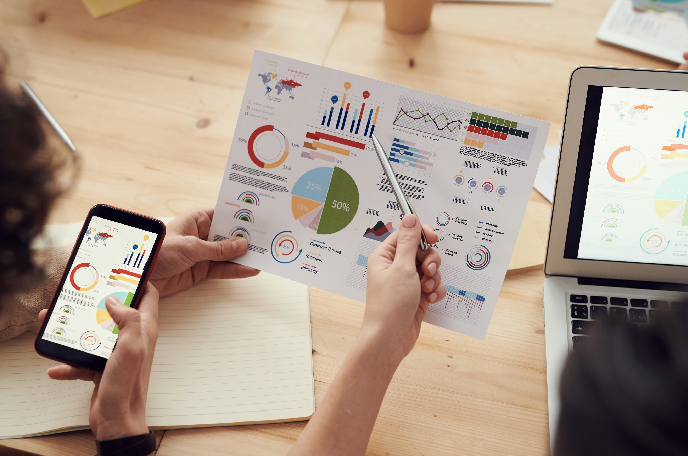
As more of our lives move online whether as consumers, students, or citizens, our various interactions are producing ever more detailed data. Businesses now have more tools to take this data to develop more efficient and personalised customer experiences. Not only in helping to design more user-friendly interfaces on a multitude of digital devices but to help to create a better overall experience through-out the customer-client journey by using data to personalise interactions with AI bots, reduce friction and so on.
5. Convergence
Over the last decade or so, much has been discussed and written about digital transformation, AI, the Internet of Things (IoT), cloud computing and super-fast networks like first 4G and then 5G. What’s new is that more and more, we are hearing about how these separately existing technologies are going to be combined allowing for even more rapid digital transformation. For example, AI will allow IoT devices to function more intelligently and to interact more efficiently without human intervention. When we couple this with ultra-fast transmission networks, we can see the roll-out of smart homes and smart workspaces and even smart cities.
6. Cloud native analytics
“Cloud-native” apps refer to software that is built to be manageable, adaptive and resilient. This type of data can be structured and stored in flexible ways so that it can be scaled up and scaled out. This characteristic provides many advantages for businesses of different sizes. Data processing and storage can be moved off site to a private or public cloud network. This is a great option for companies that have changing analytics needs and that do not want or cannot afford an on-site data storage solution.
Cloud analytics is a type of analytics model that moves data processing and storage operations to a private or public cloud network. The model is preferable for companies that have variable analytics needs and cannot afford, or wish to avoid, an on-premises data storage solution.
7. Rise of predictive analytics
Predictive analytics uses statistical modelling and advanced ML techniques to develop insights about future events. Using both historical and current data, predictive analytics builds dedicated tools and data models to forecast the future, and analyse risks and opportunities. In some cases, this type of analytics can even automate certain aspects of the business decision process eliminating inefficiencies, for example, by reducing machine down-time.
Want to see how these data analytics trends can benefit your business? — Find a data analyst for your business on Pangaea X.
Get your data results fast and accelerate your business performance with the insights you need today.